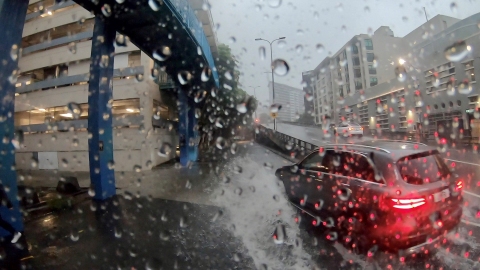
This research project aims to establish connections between weather and river flow forecasting, inundation prediction, and the associated risks to people and assets, using the RiskScape platform.
Its objective is to better understand and forecast potential impacts from extreme weather events and provide richer information to support effective decision making for Aotearoa New Zealand.
The initial focus will be on flooding as a hazard and researchers will engage with emergency managers and relevant staff in regional and local councils.
Workstream one: Weather forecasting
- Improve representation of complex terrain in NIWA’s weather models to better capture the impact of topography on rainfall.
- Verify the skill and value of new AI (artificial intelligence)-driven Neural Weather Models (NWMs) over New Zealand. While data-driven weather models have made significant advancements globally, their applicability and operational challenges in a New Zealand context remain unverified. The project will develop a 20-year ensemble hindcast of NWMs, followed by validation to build confidence in the modelling system.
- Semi automate process of running a sub-km weather model ahead of extreme events. NIWA has existing capability to run sub-km domains. We will develop automatic systems to identify potential extreme weather in our deterministic and ensemble output and (semi-) automatically run the appropriate sub-km domain.
Workstream two: Hydrology and inundation forecasting
- Design and implement an operational ensemble river forecast suite based on a dynamical weather ensemble (NZEns).
- Improve river flood forecasts by creating a post-processing river forecast method that uses statistical techniques and machine learning. A hindcast of ensemble river forecasts for the previous years will be generated to produce training and testing datasets.
- Enhance a machine-learning approach to interpolate a library of look-up scenario flood depth and extent maps for rapid inundation ensemble forecasts. This will involve designing and implementing an ensemble inundation forecasting suite to produce ensemble inundation flood depth and extent maps.
Workstream three: Hazard and risk forecasting
- Design flood impact models to estimate impacts to people and property. For example, physical damage, threats to human safety, disruption.
- Create real time property exposure workflow, connecting LINZ exposure information to flood impact models.
- Implement a pilot flood impact forecast. The case study will focus on Westport and use ensemble maps.
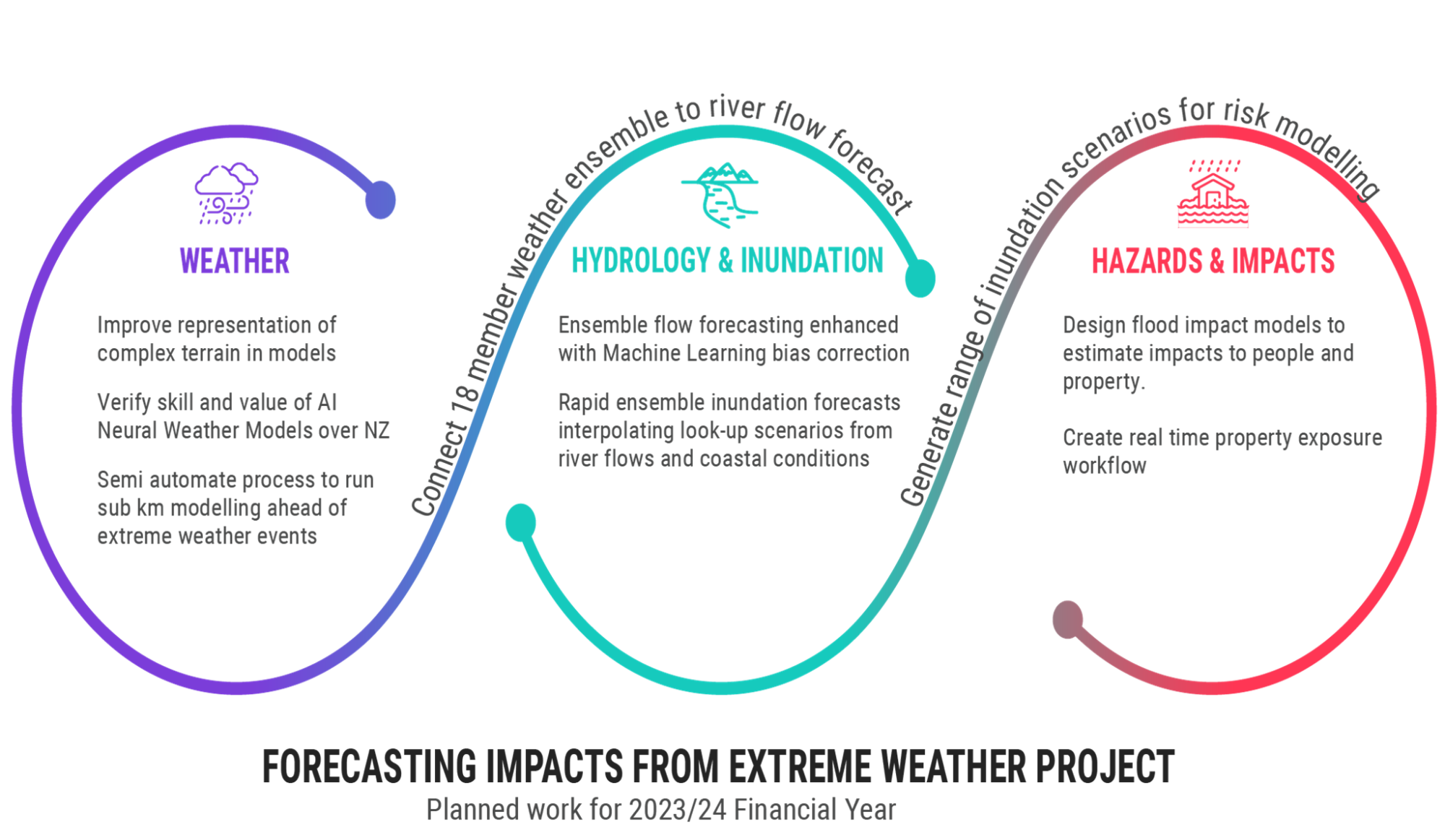